Educational Trajectories and Place-Based Disadvantage: Looking Deeper and Wider
Unequal educational outcomes are a key concern for the Government – relating to wider issues with the UK economy, social mobility and social justice. The Urban Big Data Centre (UBDC) is examining the drivers of place-based educational inequalities in order to inform educational and urban policy. In this blog, UBDC Research Associate Dr Phil Mason, part of the ‘Neighbourhoods, housing and educational opportunity‘ project, explains how linked big data can provide clarity on the surprisingly complex causes of these disadvantages.

It is often tempting to believe that, by and large, we understand the causes of many of society’s problems and that it is a short step from understanding to developing effective policies to change things for the better.
For instance, it comes as no surprise to find that if you come from a deprived neighbourhood – all other things being equal – your chances of educational success are less than those of someone from a more flourishing area. This is just common sense, after all. Or is it?
This general trend is well established by a considerable body of research from many countries and has driven the development of education policy for decades. In Scotland, for example, this inequality – the educational attainment gap – is addressed by policies such as those described in the Scottish Government’s 2017 National Improvement Framework and Improvement Plan for Scottish Education (PDF 1.4MB).
Nevertheless, such broad-brush research findings mask more complex, nuanced relationships arising from subtler, more specific causes. Until recently, limitations with the existence and availability of data have prevented these aspects from being examined in the detail we would wish to.
In the age of big data, we can now look at things in deeper and wider ways: deeper, by examining information at the finest of scales —the social-scientific equivalent of physics’ fundamental particles of matter— rather than at some level of aggregation; wider, by investigating how things change over time. Added to that, the sheer quantity of data means we can analyse many more putatively influential factors simultaneously and still be able to draw statistically reliable conclusions with confidence.
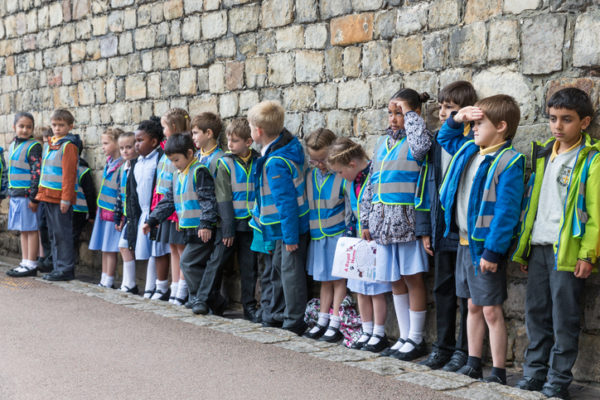
In our case, as part of the UBDC’s Educational Disadvantage and Place Project, we are using ScotXed’s secondary school data to examine in the greatest possible depth the associations between place —the pupil’s residential neighbourhood and their school— and educational outcomes, by subject, of all S4, S5 and S6 pupils studying in the Greater Glasgow local authority areas (Glasgow City, East and West Dunbartonshire, North and South Lanarkshire, Renfrewshire, East Renfrewshire, and Inverclyde). We have augmented this dataset by linking area-based measures of deprivation (SIMD vigintiles) and urban-rural location, and by calculating the distance as the crow flies between pupils’ homes and their schools. The breadth of the research arises from being able to analyse these characteristics for each school year from 2006-7 to 2014-5, so we can look at period trends and cohort effects.
The associations of pupils’ personal characteristics, their backgrounds (tagged locational data, area deprivation, urban versus rural location, distance travelled to school, etc.) and school characteristics (subject availability and choice, staffing levels etc.) with educational outcomes and subsequent educational and employment destinations have been investigated before, to varying extents. However, we have the opportunity to examine them simultaneously and over time, using powerful multivariate, multilevel statistical modelling methods.
Even these complex analyses of educational outcomes cover only a part of students’ overall educational careers and their entry (or not) into employment. Adopting a similar approach, we will extend this work using individual student-level datasets from SFC and HESA to look at the links between place and inequalities in Further and Higher Education outcomes.
We also hope to link the educational data with other datasets related to the availability of public transport, as it has been many years since the influence of available transport on participation in education in Scotland has been studied in the depth it deserves.
In this way, we hope to gain a clearer, combined perspective on the drivers of educational disadvantage throughout Secondary, Further and Higher Education and entry into employment.
By looking deeper and wider, we are sure to be surprised by many of our results: not everything turns out to be what common sense might lead us to expect.
This blog was originally posted on the UBDC website.